AI Methods for Rapid Automated Staining and Destaining of Tissue Biopsies in Hospitals
General Overview
Staining of tissues sections using chemical and biological dyes has been used for over a century for visualizing various tissue types and morphologic changes associated with contemporary cancer diagnosis. The staining procedure however is labor intensive, needs trained technicians, costly, and often results in loss of irreplaceable specimen and delays diagnoses. In collaboration with Brigham and Women’s Hospital (Boston, MA), we describe a “computational staining” approach to digitally stain photographs of unstained tissue biopsies with Haematoxylin and Eosin (H&E) dyes to diagnose cancer.
Our method uses neural networks to rapidly stain photographs of non-stained tissues, providing physicians timely information about the anatomy and structure of the tissue. We also report a “computational destaining” algorithm that can remove dyes and stains from photographs of previously stained tissues, allowing reuse of patient samples.
These methods and neural networks assist physicians and patients by novel computational processes at the point-of-care, which can integrate seamlessly into clinical workflows in hospitals all over the world.
-
Why is this work important?
Histopathology involves the visual examination of the structure and morphology of a stained biopsy tissue section under a microscope by a pathologist for diagnosis of various abnormalities. A histopathological analysis following hematoxylin and eosin (H&E) staining is considered the gold standard for the diagnosis for the majority of cancer types in liver, prostate, lung, kidney and other organs, and a variety of other diseases in humans and model systems used for biomedical research. H&E staining of tissue biopsies and subsequent visual examination by pathologists present several challenges such as variability and inconsistencies introduced by tissue preparation and staining protocols, human errors and also requires significant processing time and costs.
-
What has been done before?
Traditional methods include staining the tissue followed by microscopic analysis by a trained pathologists. Previous published methods for automated digital staining use time-consuming and expensive multispectral and hyperspectral systems for imaging the tissue, and lack clinical validation of the digitally stained images at the point-of-care.
-
What are our key contributions?
We tackle the problems of digital H&E staining of non-stained paraffin-embedded WSRI to circumvent the manual staining process, and digital H&E destaining of stained images. To our knowledge, we are the first to report digital staining and destaining of existing low-cost RGB slide images at the point-of-care. We also devise a novel loss function that enforces tissue structure preservation in GAN outputs. Our machine learning models does not require hyperspectral or multispectral imaging systems or additional biochemical steps. Microscopic morphological and sub-cellular structures in the H&E stained and destained images of paraffin embedded prostate core biopsy samples were preserved making them practical for tumor diagnosis.
-
What are the next steps?
We are actively investigating the use of our computational stained H & E images for tumor diagnosis.
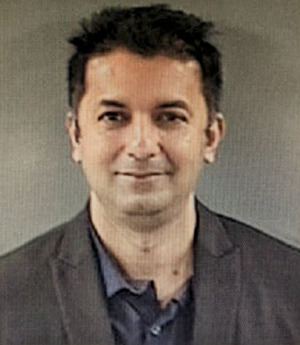
Dr. Pratik Shah
Faculty Member
Other Contributors
Alarice Lowe. Stanford University.
A. Rana, G. Yauney, A. Lowe and P. Shah, “Computational Histological Staining and Destaining of Prostate Core Biopsy RGB Images with Generative Adversarial Neural Networks,” 2018 17th IEEE International Conference on Machine Learning and Applications (ICMLA), Orlando, FL, 2018, pp. 828-834, doi: 10.1109/ICMLA.2018.00133.