Generative Deep Learning for Medical Images
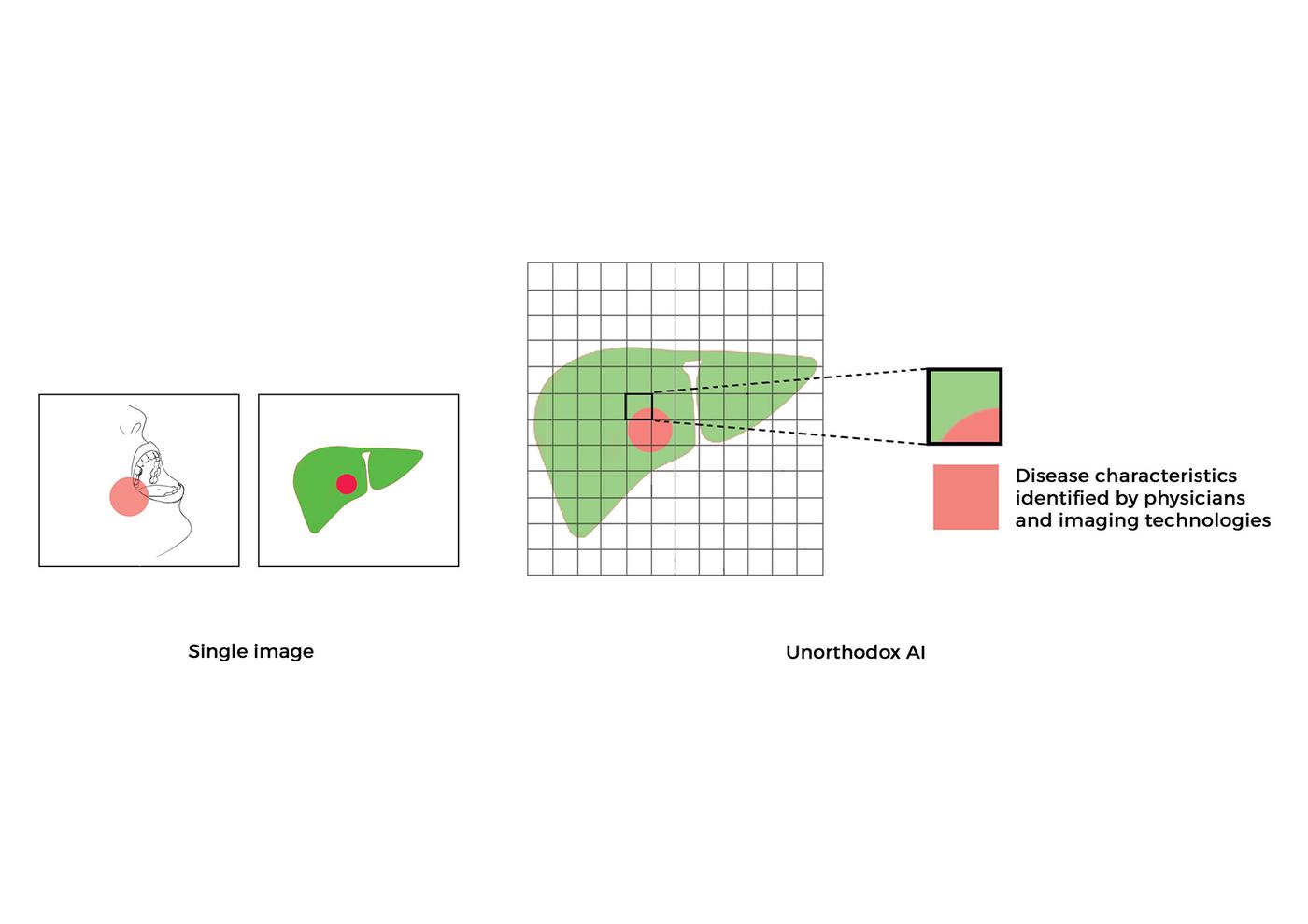
Research led by Dr. Shah and his laboratory has developed new paradigms for utilizing low-cost images captured through simple optical methods, making point-of-care clinical diagnosis more accessible and reducing reliance on specialized medical imaging devices, as well as complex biological and chemical processes. Dr. Shah’s lab has created interpretable systems and methods for the clinical translation of generative, predictive, and classification deep learning AI algorithms that extract diagnostic information from cells, tissues, and organs.
Example Projects and Collaborations:
- Advances in Uncertainty Quantification for Medical Image Segmentation: Two studies presented at the 2024 IEEE International Conference on Machine Learning and Applications (ICMLA), which focus on uncertainty quantification in deep learning models (DLMs) for skin cancer and prostate cancer image segmentation. Project 1: “Uncertainty Quantified Deep Learning and Regression Analysis Framework for Image Segmentation of Skin Cancer Lesions” introduces a novel framework that integrates pixel-by-pixel uncertainty estimation into skin cancer lesion segmentation. This method helps clinicians identify regions requiring further review, increasing trust in AI models for clinical decision making. Project 2 “Deep Learning with Uncertainty Quantification for Predicting the Segmentation Dice Coefficient of Prostate Cancer Biopsy Images” describes uncertainty quantification for prostate cancer biopsy image segmentation, predicting Dice coefficients and helping clinicians assess model performance at the image sub-region level. This study demonstrates how uncertainty maps can serve as a more reliable evaluation metric for clinical deployment. (Project and publications link).
- Workshop Paper and Special Session on Responsible Deep Learning for Software as a Medical Device: Dr. Shah conceptualized and led a special session at the IEEE International Symposium on Biomedical Imaging (ISBI 2022) focused on diversity, equity, and inclusion in medical imaging for uncertainty quantification and fair deep learning. This session featured experts from UCSF, the US FDA, and Dr. Shah, discussing the integration of unbiased imaging data, including race and ethnicity representation, into deep learning models to improve their fairness and clinical applicability. The workshop also highlighted strategies for developing high-performance, adaptable models that account for uncertainty and their regulatory pathways for real-world medical use. The extended paper, published on arXiv, explores these topics in-depth, detailing performance evaluations of AI/ML models in skin (RGB), digital pathology, and medical imaging (skin, kidneys, and prostate). The research aims to ensure safe and equitable deployment of AI-driven diagnostic tools (Paper on arXiv, IEEE Special session, Session Talk Video, Project Page)
- Improving Generalizability and Interpretation of Deep Learning Models: Deep learning models for segmenting complex patterns from medical images often suffer from poor generalizability. A study published in Cell Reports Methods presented an end-to-end toolkit that improves the generalizability and transparency of clinical-grade deep learning models. This toolkit helps researchers and clinicians identify hidden patterns in images and address issues related to the under-specification of clinical labels, reducing false positives and negatives in high-dimensional systems. While the study focused on medical images, the methods also apply to general image segmentation tasks, with wide applications in biomedical research and regulatory science (Project and publication link).
- Computational Haematoxylin and Eosin (H-&-E) Dye Staining and Destaining of Images of Tissue Biopsies: In collaboration with Brigham and Women’s Hospital, Dr. Shah’s team developed a “Computational H-&-E Dye Staining” system to digitally apply H-&-E dyes to photographs of unstained tissue biopsies for cancer diagnosis. The research also introduced a “Computational Destaining” algorithm, which removes dyes from stained tissue images, allowing reuse of biopsy samples. This innovative system uses neural networks to enhance the efficiency of diagnosing cancer while saving valuable patient samples (Project and publication link).
- Generative AI for Staining and Destaining of Images of Tissue Biopsies: A collaboration with Stanford University and Harvard Medical School led to breakthroughs in high-fidelity, explainable computational staining of tissue samples. The research focused on deep learning models that accurately “stain” non-stained tissue images to resemble computational H-&-E dye staining, and developed a novel loss function that preserves tissue structure. The models were shown to generalize well to unseen data, enabling accurate staining of patient samples and tumor grades not part of the training data. Additionally, neural activation maps were generated to enhance explainability, offering insights into the decision-making process of these models. This research was evaluated by pathologists for prostate cancer diagnosis and published in JAMA Network and covered by MIT News, demonstrating the clinical utility of virtual staining in decision-making (Project and publication link, MIT News story).
- Tumor Localization and Classification Using Computational H-&-E Dye Stained Images: Building upon the generative AI-based staining methods developed in previous work, Dr. Shah’s lab extended the use of computational H-&-E dye stained images for tumor localization and classification. In this project, non-stained biopsy images were first processed through generative models to create virtual H-&-E stained images, which were then classified using a ResNet-18 deep learning classifier to identify tumor regions. A gradient backpropagation (GBP) algorithm was employed to further localize tumor regions on the generated images, producing saliency maps that highlight areas of interest for clinicians. This research was selected for a Selected for a deep-dive spotlight session at the International Society for Optics and Photonics (SPIE) medical imaging conference for demonstrating how the computational H-&-E dye staining technique can be seamlessly integrated with tumor detection workflows, improving diagnostic accuracy, and extending the utility of virtual staining methods for real-time clinical applications (Publication link).
- Sepsis Diagnosis Using Dark Field Imaging: In collaboration with Beth Israel Deaconess Medical Center, Dr. Shah’s research explored the use of dark field imaging of capillary beds under the tongue to diagnose sepsis (bloodstream infection). A neural network developed by the lab achieved over 90% accuracy in distinguishing between septic and non-septic patients, providing a rapid method to assess sepsis and reduce unnecessary antibiotic use, helping to combat antimicrobial resistance (Project and publication link).
- Fluorescent Porphyrin Biomarkers for Cancer and Periodontal Disease: Dr. Shah led studies showing that fluorescent porphyrin biomarkers, which are typically used for imaging tumors and periodontal diseases, can be successfully predicted from standard white-light photographs. This method eliminates the need for specialized fluorescent imaging at the point-of-care, making diagnosis more affordable and accessible (Project and publication link).
- Automated Segmentation of Oral Diseases: In another study, Dr. Shah’s lab trained neural networks to automatically segment oral diseases from standard white-light photographs. These automated systems also correlate disease patterns with systemic health conditions, such as optic nerve abnormalities, to generate personalized risk scores for patients.(Project and publication link)
These examples show Dr. Shah’s lab’s contributions to the next generation of computational medicine, particularly in the development of generative AI models for medical imaging. Our goal is to assist physicians and patients in making more accurate, efficient, safe and cost-effective diagnoses at the point of care..
2024
Uncertainty quantified deep learning and regression analysis framework for image segmentation of skin cancer lesions
El Houcine El Fatimi, Shah P\*
( *Senior author supervising research)
23rd IEEE International Conference of Machine Learning and Applications. DOI: 10.1109/ICMLA61862.2024.00080
Read More >>2024
Deep learning with uncertainty quantification for predicting the segmentation dice coefficient of prostate cancer biopsy images
Audrey Xie, El Fatimi E, Ghosal S, Shah P\*
( *Senior author supervising research)
23rd IEEE International Conference of Machine Learning and Applications. DOI: 10.1109/ICMLA61862.2024.00178
Read More >>2023
Responsible deep learning for software as a medical device
Pratik Shah\*, Lester J, Delfino J, Pai V
(*Senior author supervising research)
arXiv:2312.13333 [eess.IV]
2021
A deep-learning toolkit for visualization and interpretation of segmented medical images
Sambuddha Ghosal and Shah P*
( *Senior author supervising research)
Cell Reports Methods 1, 100107, 2021
Read More >>2021
Uncertainty quantified deep learning for predicting dice coefficient of digital histopathology image segmentation
Sambuddha Ghosal, Xie A, Shah P\*
( *Senior author supervising research)
arXiv:2011.05791 [stat.ML]
2018
Computational histological staining and destaining of prostate core biopsy RGB images with generative adversarial neural networks
Aman Rana, Yauney G, Lowe A, Shah P*
(*Senior author supervising research)
17th IEEE International Conference of Machine Learning and Applications. DOI: 10.1109/ICMLA.2018.00133
Read More >>2020
Use of deep learning to develop and analyze computational hematoxylin and eosin staining of prostate core biopsy images for tumor diagnosis
Aman Rana, Lowe A, Lithgow M, Horback K, Janovitz T, Da Silva A, Tsai H, Shanmugam V, Bayat A, Shah P*
(*Senior author supervising research)
JAMA Network. DOI: 10.1001/jamanetworkopen.2020.5111
Read More >>2021
Automated end-to-end deep learning framework for classification and tumor localization from native non-stained pathology images
Akram Bayat, Anderson C, Shah P*♯
(*Senior author supervising research, ♯Selected for Deep-dive spotlight session)
SPIE Proceedings. DOI: 10.1117/12.2582303
2018
Machine learning algorithms for classification of microcirculation images from septic and non-septic patients
Perikumar Javia, Rana A, Shapiro NI, Shah P*
(*Senior author supervising research)
17th IEEE International Conference of Machine Learning and Applications. DOI: 10.1109/ICMLA.2018.00097
Read More >>2017
Convolutional neural network for combined classification of fluorescent biomarkers and expert annotations using white light images
Gregory Yauney, Angelino K, Edlund D, Shah P*♯
(*Senior author supervising research, ♯Selected for oral presentation)
17th annual IEEE International Conference on BioInformatics and BioEngineering. DOI: 10.1109/BIBE.2017.00-37
Read More >>2017
Automated segmentation of gingival diseases from oral images
Aman Rana, Yauney G, Wong L, Muftu A, Shah P*
(*Senior author supervising research)
IEEE-NIH 2017 Special Topics Conference on Healthcare Innovations and Point-of-Care Technologies. DOI: 10.1109/HIC.2017.8227605
Read More >>2019
Automated process incorporating machine learning segmentation and correlation of oral diseases with systemic health
Gregory Yauney, Rana A, Javia P, Wong L, Muftu A, Shah P*
(*Senior author supervising research)
41st IEEE International Engineering in Medicine and Biology Conference. DOI: 10.1109/EMBC.2019.8857965
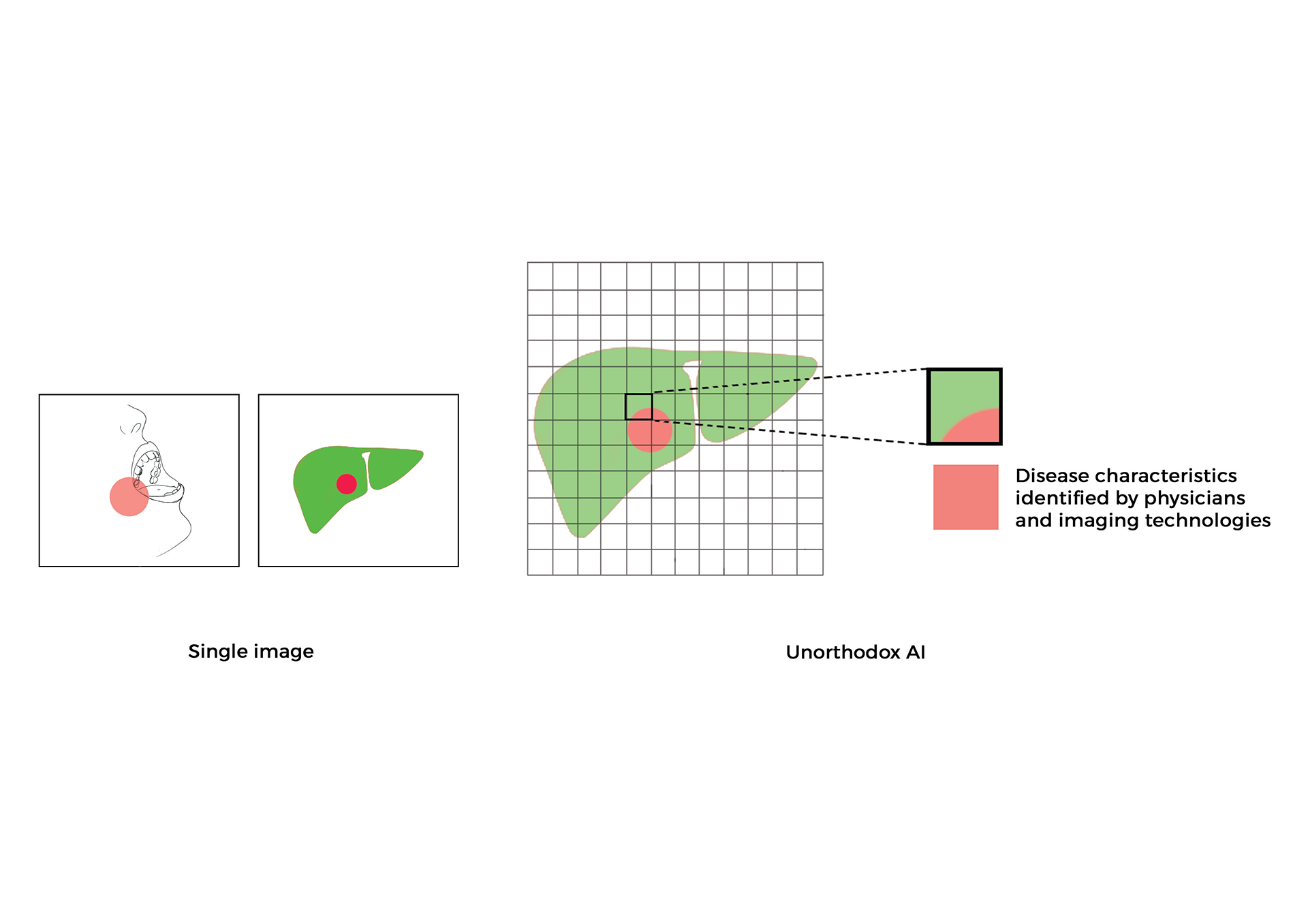
Select Talks
- 2024 - Generative AI for computational pathology
- 2022 - Biomedical imaging for equitable deep learning, regulatory science, and clinical research
- 2021 - Interpretable AI and Explainable AI
- 2020 - Novel deep learning systems for oncology imaging, digital medicines and real world data
- 2019 - Understanding biomarker science: from molecules to images
- 2019 - Unorthodox AI and machine learning use cases and future of clinical medicine
- 2019 - Machine learning methods for biomedical image analysis
- 2018 - Novel machine learning and pragmatic computational medicine approaches to improve health outcomes for patients
- 2018 - TED Talk - How AI is making it easier to diagnose disease
- 2017 - Artificial intelligence for medical images
Press
- 2020 - Deep learning accurately stains digital biopsy slides
- 2017 - New visions for the world we know: Notes from an early morning of TED Fellows talks
- 2017 - Innovation and Inspiration From TEDGlobal 2017
- 2017 - TED: Phones and drones transforming healthcare
Honors
- 2017 - TED Fellow
National Science Foundation invites Pratik Shah as Expert Reviewer
January, 10 2025 - January, 10 2025
Alexandria, VA
Pratik Shah invited to speak at the 2024 Annual Meeting of American Society for Investigative Pathology
April 23, 2024
Baltimore, MD
National Institutes of Health invites Pratik Shah as SBIR STTR Transfer study section expert grant reviewer
March 14, 2024 - March 15, 2024
Bethesda, MD
Pratik Shah invited to speak at the 2023 UCI Systems Biology Retreat
April 2, 2023
Los Angeles, CA
Pratik Shah invited to chair IEEE ISBI conference oral session on Clinician Decision Support
March 30, 2022 - March 31, 2022
Pratik Shah invited to speak at "Bringing AI to the bedside" conference hosted by National Cancer Institute and Purdue University
April 22, 2021 - April 23, 2021
West Lafayette, IN
Pratik Shah invited to speak at the Stanford Food and Drug Administration-Project Data Sphere Symposium VIII
November 11, 2019
Stanford, CA
Pratik Shah invited to speak at Elsevier conference AI and Big Data in Cancer: From Innovation to Impact
November 15, 2020 - November 17, 2020
Boston, MA
Pratik Shah invited to speak at United States FDA & UCSF-Stanford Center of Excellence in Regulatory Science and Innovation Workshop
October 1, 2020 - October 2, 2020
Washington, DC
National Cancer Institute Invites Dr. Pratik Shah as Expert Reviewer
December 9, 2021
Bethesda, MD
Pratik Shah invited to the National Institutes of Health as a Study Section Member and Grant Reviewer for Health Informatics Applications
June 25, 2020 - June 26, 2020
Bethesda, MD
Center for Scientific Review at National Institutes of Health Invites Dr. Pratik Shah to Health Informatics Study Section
November 19, 2020 — November 20, 2020
Bethesda, MD
Center for Scientific Review at National Institutes of Health Invites Dr. Pratik Shah to Health Informatics Study Section (2)
March 18, 2021
Bethesda, MD
Pratik Shah invited to speak at AI in Medicine Series, University of Texas Southwest Medical Center
September 5, 2019
Dallas, TX
Pratik Shah invited to speak at The ethics and governance of Artificial Intelligence: MAS.S64
February 3, 2018
Cambridge, MA
Center for Scientific Review at National Institutes of Health Invites Dr. Pratik Shah to Health Informatics Study Section-(3)
June 24, 2021
Bethesda, MD
Pratik Shah @ TED2020
At TED2020, we're inviting you on a bold voyage into uncharted territory.
May 18, 2020 - July 10, 2020
Vancouver, Canada
Two publications from Dr. Pratik Shah's lab at IEEE ICMLA
December 18, 2018 - December 19, 2018
Orlando, FL
Pratik Shah invited to speak at View Conference 2016
October 24, 2016 - October 28, 2016
Turin, Italy